AI Content for Journalism: Unleash it, or control it?
Artificial intelligence ought to help journalists and other content creators. It’s modern and efficient. Feed it data, tell it the subjects you want covered and watch as the finished articles pop up on your screen. Make a few tweaks and send them out. Sit back and watch the profits come in.
What could go wrong?
Everything — at least when you’re expecting AI to create reader-ready content.
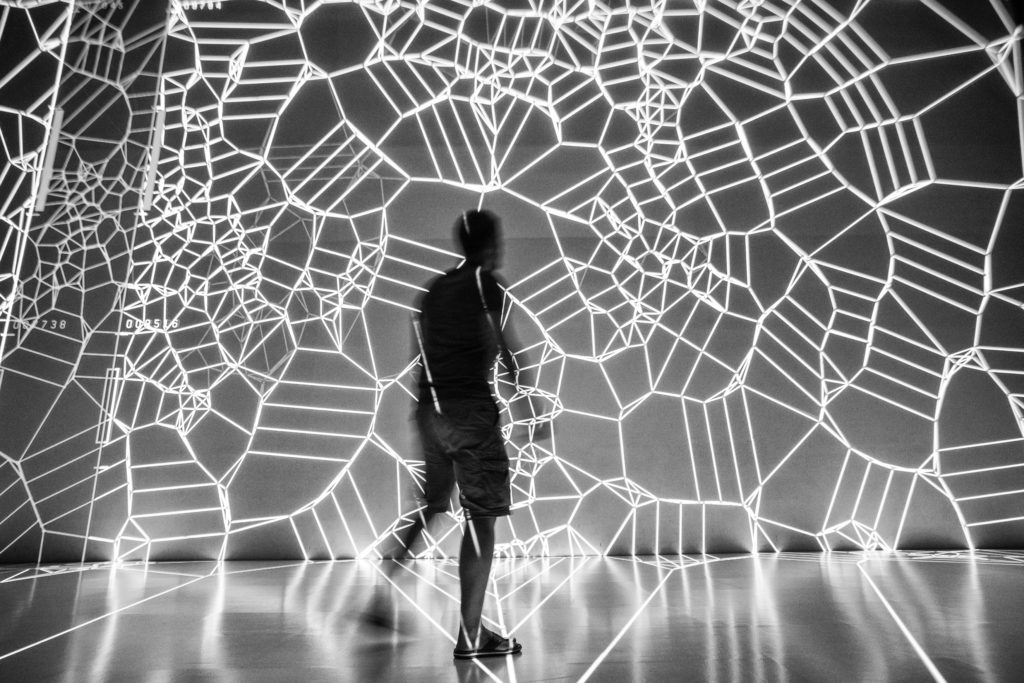
AI today, and in the foreseeable future, works best as a tool for creating simple stories and drafts. It’s a bit like a student research assistant but one that doesn’t get hungover, doesn’t need coffee and doesn’t complain about minimum wage. AI-drafted content will need to be scrutinized and fixed up, just like that of the student researcher. When used in this intermediary/research manner, AI will probably live up to expectations, providing useful and efficient preliminary research and first drafts.
But sending AI-created content directly out to news consumers? Don’t even think about it, given the many deficiencies and biases inherent in AI-created content.
Some of the problems with AI-created content have been recognized. In a widely reported 2022 paper, several researchers, including one previously at Google, warned of “the risk of substantial harms, including stereotyping, denigration, increases in extremist ideology, and wrongful arrest” associated with AI content creation. So the special case of journalistic use of AI-developed content deserves careful study.
AI-created content comes from a limited fixed universe — existing digital content. That’s quite different from content created by a human reporter. (Let’s call her Lois Lane of the Metropolis Daily Planet.)
When Lois Lane goes out on a story, she drives the streets, walks the neighborhoods, knocks on doors and talks to people who’ve witnessed an event. She checks with officials and civic leaders and inspects the place where the events occurred. Through this customized fieldwork, Lane finds new information.
AI programs, by contrast, draw solely from digital text, both when they are taught, and when they create content. Like the dots and lines in two-dimensional Flatland, the AI program can’t imagine our 3-D world. AI works solely with information in digital databases.
The digital text that’s used to train AI programs is limited and biased (even if it is accurate.) Most of the content was created in the last few decades, disproportionately by English-speaking people of wealth and power. Much was created for advocacy or polemical purposes. There’s lots of sloppy social media and Internet content; by contrast, because of copyright, permission, and availability issues, they will likely be very light on the content of current published books carefully written by experts. This narrowness creates the database bias of AI content. (Database bias isn’t new; libraries and publishers frequently overemphasize certain subjects, like war. AI is like a reader who digests all of the books in the library and comes out knowing far more about war-making than peacemaking.)
Human mistakes can enter when machines are taught to use the databases. Computer algorithms are simply sets of instructions for solving a problem, or set of problems, or meeting an objective. Human algorithm writers necessarily contribute some programmer bias.
There’s more. AI excludes live witnesses and real-world settings, and basic background understandings of human nature and human communities. Lois Lane does fieldwork; AI never does. AI programs miss context and real-world understandings (things that even that sleepy student research assistant might include). These omissions make up the incomplete picture bias of AI-created content.
Then there is simple accuracy. AI tools make mistakes. Consider the AI translation program that inaccurately translated a Palestinian’s “good morning” into “hurt them” in Hebrew. Or the early days of Google News, when it at times featured Onion parodies as top breaking news stories. This is erroneous content creation. The effect of such errors depends somewhat on the audience. Will readers look skeptically at AI-created content, and apply good media literacy analytical skills? Or might readers trust AI, thinking it will eliminate human judgments and biases?
Next we have to consider that today’s digital data contains lots of disinformation. Disinformation purveyors disguise their identities, hide their tracks, and employ psychologically sophisticated persuasion techniques, so their materials permeate the Internet, and can’t be easily detected. Even readers skilled in media literacy often struggle to separate out reliable from unreliable information online. AI programs, which have no moral compass of their own, will be fed lots of disinformation, which will work its way into the AI process. This is disinformation bias.
Moreover, AI systems may well perpetuate and promote disinformation. That’s certainly occurred with content-selection algorithms used by Facebook and YouTube. Algorithms can’t make moral judgments. Current social media algorithms rely a lot on user choices and preferences, meaning that they often promote high-emotion content, the stuff that gets lots of hits and reposts. Some high emotion content is innocuous, like cat videos, but much more of it is hate and invective. This interplays with disinformation; impulsive social media users often embrace and repost disinformation and hate. AI content-creation programs may well mistake disinformation content’s many hits and reposts as markers of credibility, and therefore use it in its own content. This would be disinformation perpetuation. One scholar who writes about “algorithmic amplification” notes, “The feedback loop is amplified by algorithms in the digital environment, which promote attitude-consistent information selection and limit cross-cutting news options,” all of which “may amplify existing fears, distrust, and confirmation bias.”
Let’s move from information gathering to writing. How will Lois Lane’s and AI’s stories differ?
When she sits down at her keyboard — assuming she’s not distracted or daydreaming — Lane thinks about the places she’s seen, the people she’s interviewed, their points-of-view and prejudices, similar past events, her own knowledge of human nature, and even community norms, myths, hopes and fears. With this background, and considering journalistic conventions and the desire for objectivity, she carefully writes what she believes will be a fair, reliable and complete account. (Of course, if there’s a soccer game that night, she may rush and cut some corners.)
Her counterpart at the Daily Planet’s competitor will similarly present his or her own picture of the event, enriched by his or her own field reporting, and reflecting his or her own background, judgments, and distractions. In the old days of news competition, readers could get a pretty full picture from reading competitive multiple accounts. So human reporting benefits from both customized fieldwork and diverse human judgments.
The AI program, by contrast, will never be distracted or hurried. It will follow its programmed design, and most likely write an organizationally and grammatically respectable report. It will begin with a clear topic sentence, report supporting data, and finish with a generally reasonable conclusion. It’ll look good, maybe even better than Lane’s human-written counterpart. But it will reflect the biases of the database, the lack of any field investigation, the lack of community understanding, and the ugly blots of relied-on disinformation. And while different AI programs will generate different stories, they’ll all carry similar machine deficiencies. That is, AI-generated content will inevitably involve drawing from limited and biased data without human judgment.
Now let’s move to the actual publication.
When Lane’s story comes out in the Daily Planet, it bears her byline: a direct attribution. Readers will know who wrote it and who to complain to. How will the AI story be credited? With no byline, but presented as news of comparable reliability and worthiness? Bearing the byline of a real or imaginary reporter? Hopefully, it will come with full explanation of its AI-based creation; without that, the publisher will risk engaging in misleading attribution, itself a deceptive technique, as it would give the AI-written story undeserved credibility.
Thus, from the viewpoint of just one story, AI content creation raises serious concerns about information bias, completeness, reliability and transparency.
Even more troubling concerns arise when we look beyond individual stories to the broader effects of a news publishing system that substantially relies on unvetted AI-created content.
Digital content isn’t itself the defining element of modern communications; what’s most special is the immediacy of publication and response, and the breadth of those who participate in both publishing and responding. If digital content only came from established publishers, was only published on fixed cycles, and contained no mechanism for immediate response, we’d essentially be back in the traditional media world. So we must ask: what will happen to AI content when it is sent out immediately and can be reposted and responded to immediately?
Social media has shown us that online posts go through cycles of immediate publication, redistribution, and responses. AI news content, even when erroneous or biased, is likely to be immediately read, responded to and redistributed. Most ordinary readers don’t carefully vet the content they see; if it comes from trusted sources or otherwise appeals, they accept it, apply their personal or tribal interpretations and then use it in their own communications. That is, even clearly mistaken AI content is likely to be immediately reposted and further disseminated by those who find it comfortable and useful.
Suppose another pandemic breaks out. An editor tells an AI tool to write about whether vaccines work. Given the earnestness of the anti-covid vaccine crowd, and the financial strength of the far-right, there’s a lot of anti-vaccine material on the Internet, and therefore in the databases used by AI. So early in the new pandemic, AI “news” reports, drawing from databases with lots of anti-vaccine content and unrestricted by editorial judgment, will certainly describe anti-vaccine arguments, and probably sow doubts about vaccines. Anti-vaccine people, with strong feelings on this subject, will actively repost these accounts, which will lead to further dissemination. This is the spiral of misinformation concern.
Finally, the automation of news creation and dissemination can exacerbate the neglect of responsibilities over published materials. Although editors make their news selections for a particular time and place of publication, most often today that content immediately becomes available worldwide, essentially forever. Even false, misleading, outdated and incomplete content stays online, and becomes searchable and useable worldwide. Automated AI-generated content could bring more worldwide no-end-set distribution of content, including misleading content. Just as the printed word carries more harm than the spoken word, because of its permanence, the never-ending worldwide distribution of false or misleading information can clearly cause great harm.
Who will review, correct or takedown, as appropriate, AI-created automatically distributed content? Even today many human editors and publishers disclaim responsibility for the content they have released forever worldwide; will machines accept any greater responsibility? This concern is one of failure to responsibly manage online content.
All the concerns outlined here, of course, relate to direct journalistic dissemination of AI-created content. This is quite different from merely using AI-created content as a research tool or a first draft. Machine content analysis can be particularly useful, reviewing and analyzing large datasets, making them into human-understandable summaries. But those who make research and first-draft use of AI content will need to understand the many biases and deficiencies of such content. Editorial supervision is never easy. The quantity and flaws of AI-created content will increase the demands of editors and other journalists — to carefully review, correct, improve and supplement AI-created content before it is published.
Finally, we can’t neglect the concern that in our technologically obsessed profit-driven society, number-crunching media owners will want to send AI-generated news reports directly to news consumers, despite the serious risks of bias, inaccuracy and perpetuation of disinformation. If that occurs, professional journalists will need to stand up and insist on a careful traditional intervening editorial process — yes, a human-judgment intense, non-technological and sometimes inefficient process — to ensure that their published news reports give readers, listeners and viewers a fair, accurate and complete picture of the world.
Like other human-machine confrontations since the dawn of the industrial age, AI-created content may present a stark question: Will we maintain traditional standards (in this case, professional journalism), or slack off on them in the name of efficiency and industrial progress?
Click here to support Gateway Journalism Review with a tax-deductible donation. (GJR was founded as the St. Louis Journalism Review.)
Mark Sableman is a partner at Thompson Coburn LLP, and a frequent contributor to GJR. He prepared this article in connection with Webster University’s 2023 Media Academy, which was held on March 8.